Multivariate Time Series with Linear State Space Structure: A Comprehensive Guide

Multivariate time series are a collection of time series that are related to each other. They are often encountered in a variety of applications, such as economics, finance, and engineering. In many cases, the relationships between the time series can be complex and nonlinear. However, in some cases, the relationships can be described by a linear state space model.
Linear state space models are a powerful tool for modeling and forecasting multivariate time series data. They are based on the idea that the observed time series are the output of a hidden state process that evolves over time. The state process is typically assumed to be linear and Gaussian.
In this article, we will provide a comprehensive overview of multivariate time series with linear state space structure. We will begin by introducing the basic concepts of state space models. We will then discuss the estimation and forecasting of state space models. Finally, we will provide some examples of applications of state space models in various fields.
5 out of 5
Language | : | English |
File size | : | 8755 KB |
Screen Reader | : | Supported |
Print length | : | 558 pages |
A state space model is a statistical model that describes the evolution of a hidden state process over time. The state process is typically assumed to be linear and Gaussian. The observed time series are then assumed to be a linear function of the state process.
The state space model can be represented by the following equations:
x_t = F x_{t-1}+ w_t y_t = H x_t + v_t
where:
- x_t is the state vector at time t
- y_t is the observed time series at time t
- F is the state transition matrix
- H is the observation matrix
- w_t is the process noise
- v_t is the observation noise
The process noise and observation noise are assumed to be independent and normally distributed.
The parameters of a state space model can be estimated using a variety of methods. The most common method is the Kalman filter. The Kalman filter is a recursive algorithm that estimates the state vector at each time point given the observed time series up to that point.
The Kalman filter can be used to estimate the parameters of a state space model in two steps. In the first step, the Kalman filter is used to estimate the state vector at each time point. In the second step, the estimated state vectors are used to estimate the parameters of the state space model.
Once the parameters of a state space model have been estimated, the model can be used to forecast the future values of the observed time series. The forecast can be obtained by using the Kalman filter to predict the state vector at future time points. The predicted state vectors can then be used to predict the future values of the observed time series.
State space models have a wide variety of applications in various fields. Some of the most common applications include:
- Economics: State space models are used to model and forecast economic time series, such as GDP, inflation, and unemployment.
- Finance: State space models are used to model and forecast financial time series, such as stock prices, interest rates, and exchange rates.
- Engineering: State space models are used to model and forecast engineering time series, such as temperature, pressure, and flow rate.
Multivariate time series with linear state space structure are a powerful tool for modeling and forecasting time series data. They are based on the idea that the observed time series are the output of a hidden state process that evolves over time. The state process is typically assumed to be linear and Gaussian.
In this article, we have provided a comprehensive overview of multivariate time series with linear state space structure. We have introduced the basic concepts of state space models, discussed the estimation and forecasting of state space models, and provided some examples of applications of state space models in various fields.
5 out of 5
Language | : | English |
File size | : | 8755 KB |
Screen Reader | : | Supported |
Print length | : | 558 pages |
Do you want to contribute by writing guest posts on this blog?
Please contact us and send us a resume of previous articles that you have written.
Best Book Source
Ebook Universe
Read Ebook Now
Digital Book Hub
Ebooks Online Stores
Fiction
Non Fiction
Romance
Mystery
Thriller
SciFi
Fantasy
Horror
Biography
Selfhelp
Business
History
Classics
Poetry
Childrens
Young Adult
Educational
Cooking
Travel
Lifestyle
Spirituality
Health
Fitness
Technology
Science
Arts
Crafts
DIY
Gardening
Petcare
David Eldridge
Drew Harvell
Peter Graham
Dan Vittorio Segre
Peter Howson
W Caleb Mcdaniel
Steven Selikoff
Tom Nolan
Rex A Sinquefield
Neal Pollack
Robert Gay
Daniel Boulud
Lora S Irish
Aidan Dodson
Carolina Maria De Jesus
Marcel Cartier
Isabel Vincent
Carlos G Groppa
Gilda Radner
Ken Mcelroy
Light bulbAdvertise smarter! Our strategic ad space ensures maximum exposure. Reserve your spot today!
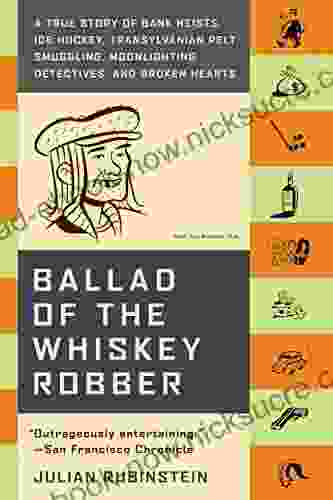

- Eugene PowellFollow ·18.6k
- Art MitchellFollow ·13.7k
- E.E. CummingsFollow ·17.7k
- Floyd RichardsonFollow ·13.1k
- José MartíFollow ·4.7k
- Mark TwainFollow ·10.2k
- Marvin HayesFollow ·9.4k
- Greg CoxFollow ·3.9k
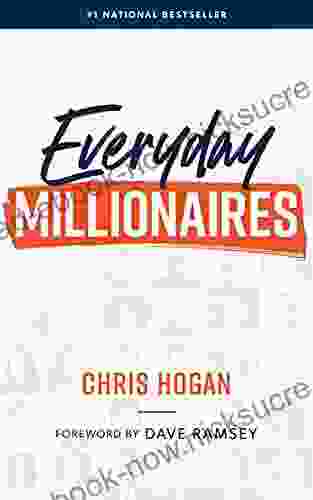

Chris Hogan: The Everyday Millionaire Who Shares His...
Chris Hogan is an Everyday Millionaire who...
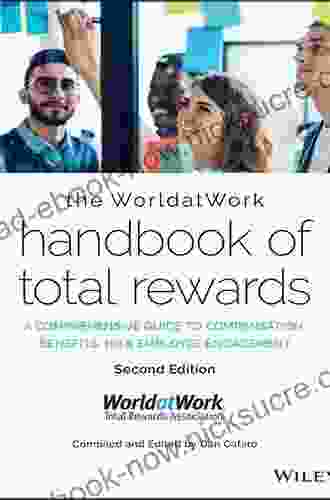

The Comprehensive Guide to Compensation, Benefits &...
In today's...
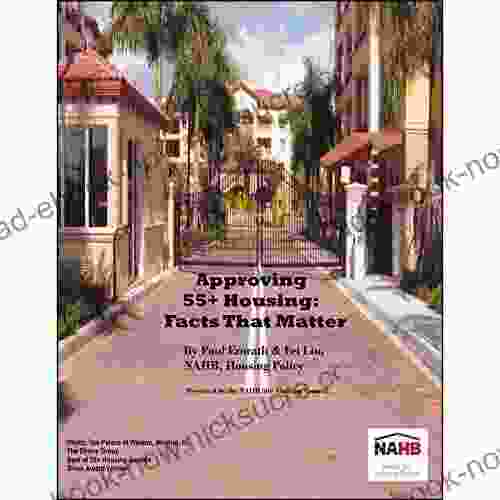

Approving 55 Housing Facts That Matter
Housing, an essential aspect...
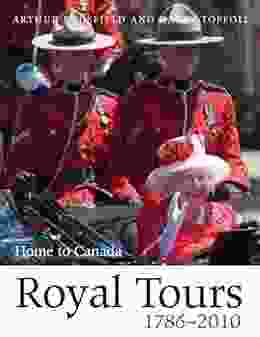

Unveiling the Enchanting Heritage of Royal Tours: A...
Canada, a land steeped in history...
5 out of 5
Language | : | English |
File size | : | 8755 KB |
Screen Reader | : | Supported |
Print length | : | 558 pages |